The Ultimate Guide to Video Labelling Tools for Enhanced Data Annotation
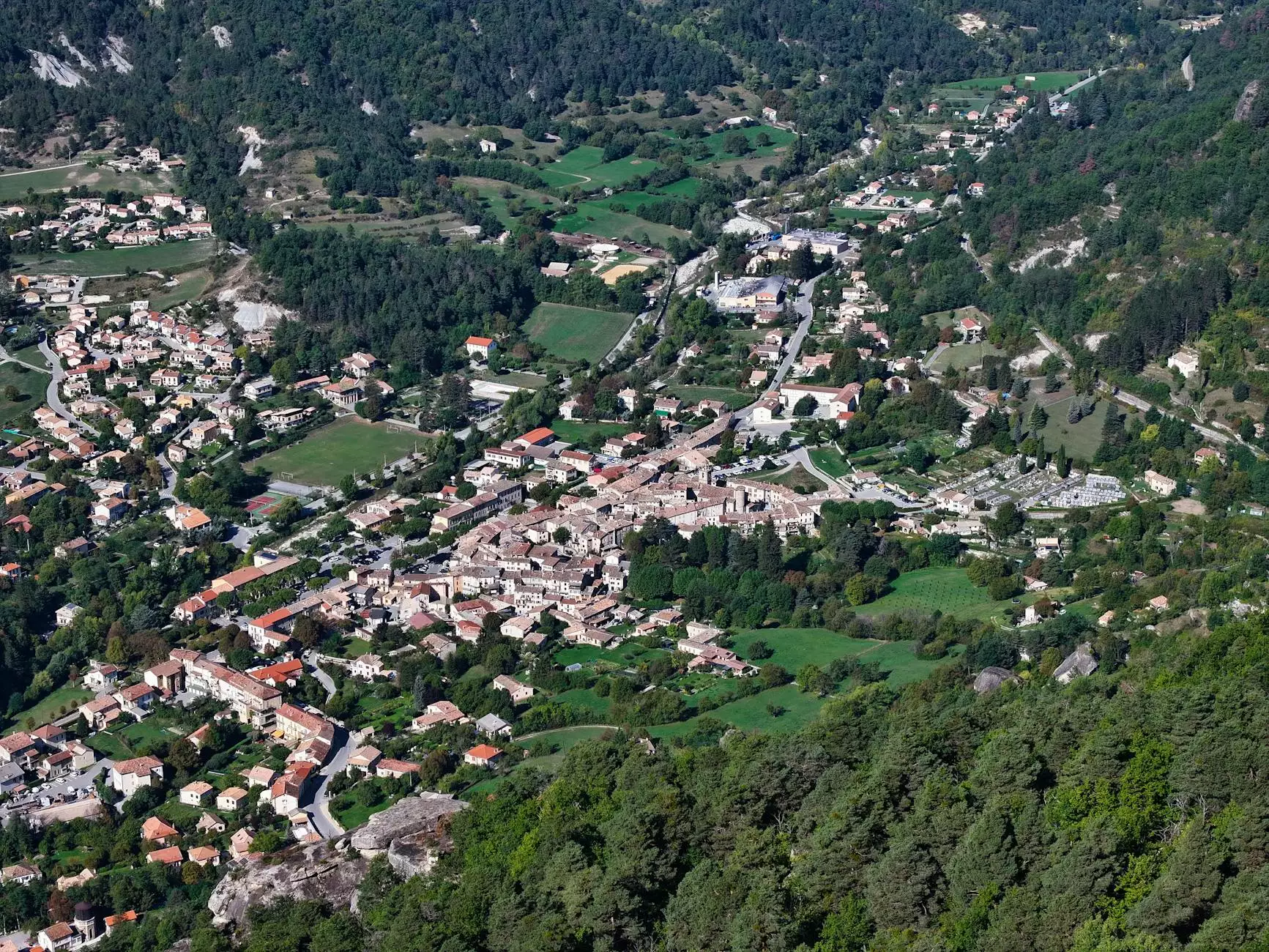
In the rapidly evolving field of technology, data annotation plays a crucial role, particularly in the areas of artificial intelligence and machine learning. Among various data types, video data has emerged as a significant contributor to the development of intelligent algorithms. This comprehensive guide will delve into the essential features and benefits of using a video labelling tool to improve your annotation projects, streamline your workflow, and enhance the accuracy of your models.
Understanding the Importance of Video Labelling
As businesses and researchers strive to utilize video data for machine learning applications, the importance of an effective video labelling tool cannot be overstated. Proper labelling is the foundation on which the success of any model is built. Here’s why video labelling is critical:
- Data Quality: Accurate labels enhance the quality of the dataset, leading to better model performance.
- Automation: Efficient labelling reduces the time spent on manual labour, allowing teams to focus on analysis.
- Scalability: As video data volumes increase, scalable solutions are essential for handling large datasets.
- Collaboration: A robust labelling tool facilitates collaboration between team members, ensuring consistent standards.
Key Features of a Comprehensive Video Labelling Tool
When selecting a video labelling tool for your projects, it’s vital to consider a set of features that will aid in achieving your goals. Here are some key attributes to look for:
1. User-Friendly Interface
A user-friendly interface enables annotators to navigate the tool with ease. This feature ensures that even those with minimal technical expertise can label videos effectively, minimizing training time and maximizing productivity.
2. Annotation Versatility
Choose a video labelling tool that supports various annotation types, such as:
- Object detection
- Image segmentation
- Action recognition
- Scene classification
This versatility allows you to cover a wide range of use cases, enhancing the applicability of your labelled data.
3. Real-Time Collaboration
In today’s interconnected world, remote collaboration is essential. A good video labelling tool should support real-time collaboration, allowing multiple users to work simultaneously. This feature encourages teamwork and can dramatically speed up the labelling process.
4. Quality Control Mechanisms
Building a high-quality dataset involves rigorous quality checks. Look for tools that provide options for:
- Review and feedback systems
- Automated quality assurance checks
- Annotation auditing capabilities
These mechanisms ensure that the labels applied are consistent and accurate throughout the dataset.
5. Integration Capabilities
Your labelling tool should seamlessly integrate with other software and platforms. This connectivity is vital for:
- Data storage solutions
- Machine learning frameworks
- Project management tools
Such integrations create a cohesive workflow that optimizes data handling and boosts overall project efficiency.
Evaluating Top Video Labelling Tools in the Market
With numerous video labelling tools available today, it can be challenging to identify the best fit for your specific needs. Below, we evaluate some top contenders based on their features, usability, and overall effectiveness.
1. KeyLabs AI Video Labelling Tool
At the forefront of video annotation technology, the KeyLabs AI video labelling tool stands out due to its robust feature set. Some key highlights include:
- Advanced Annotation Techniques: Supports a variety of labels, including bounding boxes, polygons, and keypoints.
- Intuitive Workflow: Allows users to annotate videos in a single, streamlined process, drastically reducing the time needed for data preparation.
- Collaboration-Friendly: Facilitates teamwork with a shared workspace that enables real-time feedback and revisions.
- Automated Labelling Features: Uses machine learning to suggest labels, significantly speeding up the annotation process.
This tool embodies the perfect combination of efficiency, accuracy, and ease of use, making it an excellent choice for businesses and researchers alike.
2. VGG Image Annotator (VIA)
The VGG Image Annotator is an open-source option that provides essential features for video labelling. Its strengths include:
- Free and Open Source: Accessible to everyone without licensing costs.
- Customizable Options: Users can tailor the tool to fit specific project requirements.
- Community Support: A thriving community contributes scripts and updates, enhancing functionality.
While VIA may not rival KeyLabs in terms of advanced features, its cost-effectiveness and versatility make it a notable alternative.
3. Supervisely
Supervisely focuses on providing an extensive ecosystem for data annotation and computer vision. Its features entail:
- Multi-Purpose Labelling: Supports both images and videos, allowing for varied datasets.
- Integration with AI Workflows: Easily integrates with popular machine learning libraries.
- Customizable Tools: Provides a suite of pre-built tools that can be adapted to various annotation needs.
Supervisely is suitable for organizations that require a comprehensive platform handling both labelling and model training.
Best Practices for Video Labelling
To ensure the success of your video labelling projects, it is essential to follow best practices. Below are some tips to help you maximize your results:
1. Define Clear Annotation Guidelines
Before starting, outline a set of guidelines to ensure consistency across all labelled data. This includes:
- Label definitions
- Examples of correct and incorrect annotations
- Best practices for handling ambiguous cases
2. Regular Training Sessions
Conduct regular training sessions for annotators to reinforce knowledge of the guidelines. This practice can significantly improve inter-annotator agreement, leading to a higher quality dataset.
3. Iterative Feedback Loops
Establish feedback loops where experienced annotators review the work of newer members. Continuous feedback helps identify issues early and aids in the development of annotators’ skills.
4. Utilize Quality Control Measures
Incorporate quality control measures such as random audits and checks to ensure that the labelling conforms to established standards. These checks help to maintain the integrity of the data over time.
Conclusion: Embrace the Future of Data Annotation with Video Labelling Tools
In summary, the role of a video labelling tool is foundational to the success of any machine learning project involving video data. By choosing the right tool, defining clear guidelines, and adhering to best practices, businesses can streamline their annotation processes and significantly improve data quality.
As the demand for high-quality labelled data continues to surge, leveraging sophisticated tools like those offered by KeyLabs AI is vital for staying competitive in the market. By integrating these solutions into your workflow, you pave the way for enhanced collaboration, efficiency, and ultimately, more accurate and reliable AI models.
For further insights and to explore our advanced annotation solutions, visit KeyLabs AI today!